College students, like other young consumers, are notoriously fickle and may not exactly seem like an ideal candidate for loyalty marketing. But there are at least two things that make college students hard to ignore for many businesses. First, college kids are usually at the cutting edge of technology and fashion trends. After all, two of the major online players today, Google and Facebook, had sprung from university campuses. This makes college students important trendsetters to watch. Second, although college kids may not be rich today and some may even have concerns paying for their education, they do tend to spend more (a good 40%) on discretionary items such as fashion and entertainment. They are also well on their way to joining the educated professional workforce in a few years. So for some businesses, college students represent valuable customers right now, and for many others, these students are potentially valuable customers in the near future.
Because of this, it is important for loyalty marketers to get into the mind of college students. Recently, I conducted a brief survey of 184 college students. Similar to an earlier study I did on adult consumers, the purpose of this survey was to understand college students’ online social activities and their consumption and sharing of online content. Some findings from this survey were expected, while a few surprising facts also emerged. Here are some highlights.
Tag: user-generated content
Rising to Stardom: What Makes Some User-Generated Content So Popular?
For the longest time, I’ve wondered what brings the extraordinary success of some user-generated content. Consider, for example, the top ten most popular YouTube videos of all time. The #1 video on the list is a simple one-minute clip of a little baby biting his British English-accented brother’s finger. But it has received a whopping 155+ million views, while your average YouTube video probably doesn’t get much more than a dozen passerby’s attention. Why such a huge difference? I asked. When I spoke with my friend Michelle Rogerson, she expressed the same curiosity. So we decided to set out to answer our question.
To do this, we collected a random sample of slightly more than 100 videos from YouTube over the course of a week. These are all fresh new videos just uploaded onto YouTube, so that we can study their rise to popularity from scratch. We traced each video for a period of two months, recording the number of views and the average user ratings each day. We also collected a large number of characteristics for each video (see the figure below), including those related to the video content, to the video author, and to the network of users connected to the video author. We further recruited a group of individuals to rate each video on its production quality, educational value, and entertainment value, which are the three components of what we call “innate content quality”.
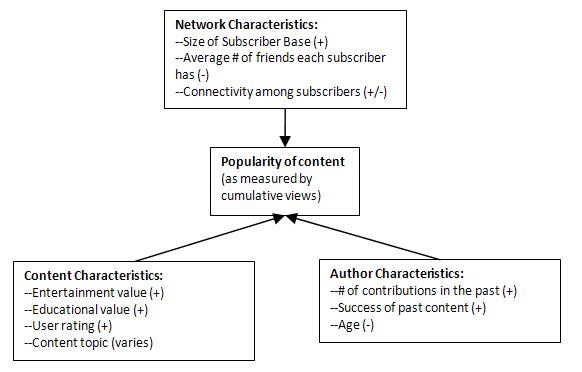
Equipped with all these data, we then used a technique called recurrent events analysis to see how these video characteristics affect the popularity of a video. Below are some of the main things we found:
- Authors with a large number of subscribers who each has only a handful of friends are in a better position than authors with a small number of subscribers who in turn may have a large number of friends.
- Once past an author’s direct network of subscribers, influence rather than simple reach becomes critical. We attribute this to the large amount of user-generated content (UGC) being passed around everyday and as a result our tendency to ignore most sharing unless they come from someone we are really close to or someone whose opinion we respect (opinion leadership is not dead!)
- What proportion of an author’s subscribers know each other also matters. A totally segregated set of subscribers does not help get the words out, but a group of subscribers where everyone knows everyone else is not optimal either. We found that the ideal connection ratio (termed connectivity or density in network analysis) was about 38%. Below this ratio, diffusion rate increases with connectivity up to the maximum, and then decreases after this threshold.
- Of the three innate quality components, entertainment value and educational value are equally important in determining a video’s popularity. Production quality, on the other hand, did not matter.
- But the biggest impact did not come from innate quality but from what we call “manifested quality”, which is quality information publicly available through other users’ ratings (i.e., the little stars underneath each YouTube video). Increasing the average rating by 1 star can lead to as much as 13.5% gain in diffusion rate.
- Age has a negative effect on diffusion rate, meaning that younger users’ contributions are more likely to be popular.
- An author’s past experience and success also carry over to the new content. More prolific authors and authors whose past contributions were more popular are more likely to see their new content popular.
Of course, with only one study, we are far from completely answering our initial question. But what we found here suggest that there are indeed systematic differences among videos and authors that can help predict the success of future content. Carrying this over to other types of user-generated content such as tweets and consumer blogs, these findings and findings from future studies should help companies pour through the overwhelming amount of user-generated content available online and selectively invest effort in the ones that are most likely to become popular.
What do you think? I’d love to hear your thoughts. Is there anything important that we are missing? If you are interested in more details about our study, you can download our working paper at http://www.yupingliu.com/files/papers/liu_rogerson_ugc_diffusion.pdf.
Wharton Conference on User-Generated Content Part II
Last week I blogged about a few research projects presented at the Wharton conference on user-generated content. In this second part of the conference summary series, I’d like to discuss one other interesting presentation that is not as directly related to user-generated content per se but I think can be of tremendous interest to online advertisers. Then to wrap up the series, I will list a few research questions raised by industry participants at the conference. This will probably be particularly interesting to researchers who are wondering what is on the practitioners’ mind. By the way, the conference has created a page with links to all the presentation slides.
http://www.flickr.com/photos/teofilo/ | CC BY 2.0
How to target ads to consumers without sacrificing their privacy?
The recent controversy surrounding Facebook’s privacy setting changes shows us that privacy issues are still very much on people’s mind these days, especially with a large amount of very personal data now available through online social networks. To advertisers, the increasing amount of social and personal information represents a great opportunity to offer very targeted ads to consumers. But as we get closer to consumers’ personal domain of interests and friend networks, advertisers are also treading a very dangerous water of consumer privacy. This is why I find New York University Professor Foster Provost’s research to be particularly interesting, as it allows target advertising toward consumers while still protecting their privacy, or in the researchers’ term, “privacy-friendly” target advertising.
The basic idea is quite simple, although the actual implementation can become more complex and mathematical. The underlying premise of the approach is that consumers who are more similar to each other are more likely to buy the same brands and share similar consumption habits. This is why social network information can be very powerful, because we are likely to buy the same things as our friends or at least have a good deal of influence on each other. The problem with using such explicit social network information is the privacy issue. To circumvent this problem, Professor Provost’s approach uses anonymized browsing data instead. It builds on two key sets of information: (1) a set of consumers who are considered brand actors; and (2) browsing data for these brand actors and other consumers whose brand affinity is not yet behaviorally demonstrated.
For the first set, one can use criterion such as having visited a brand’s website or fan page on Facebook to identify consumers who are brand actors. Notice that advertisers do not need to know who these consumers actually are in terms of names or demographics, but just that they are entities who have demonstrated certain desired behavior. Then with this information, the brand proximity/affinity of other consumers can be calculated by analyzing how closely the content (brand and non-brand related) visited by those consumers resemble that of the brand actors. Potential consumers can then be ranked based on this similarity to identify the ones that have the closest brand proximity. Professor Provost’s research shows that consumers picked in such a fashion have a much higher concentration of potential brand actors than random picking and that these consumers are much more likely to be linked to known brand actors. A paper from this research project is available from Professor Provost’s website.
To me, the beauty of this research is two-fold. First, because the only data needed are browsing logs without personally identifiable information attached, it allows advertisers to selectively target consumers without having to worry about privacy issues. Second, because the approach is defined in a sufficiently general fashion, it allows for much tweaking and customization. For instance, various brand proximity measures can be used (this research itself suggests five measures), and different measures can be combined to most accurately gauge brand affinity. Moreover, the criteria used to spot brand actors can be customized based on an advertiser’s needs (e.g., visit to awareness page vs. conversion page depending on the goal of the campaign). Such flexibility makes the approach applicable to a wide variety of situations.
What do practitioners want to know?
The conference organized a few industry panels to talk about their own experiences and their unanswered questions. Out of these industry participants, Mr. Gary Spangler, E-Marketing Manager from Dupont, spoke the most systematically about a set of research questions that need to be addressed from a practitioner’s standpoint. Many of these questions were echoed by other industry participants. I list them here for the benefit of academics who are in search of practically relevant research questions.
- There are more and more ways to reach/touch consumers. Is there a way to analyze the value of each electronic touch (e.g., email, social network, etc.)?
- When lead time is relatively long (e.g., 1 year or more in the case of B2B marketing), how does one measure the ROI of online marketing investment? (We all know that ROI has always been an issue, but longer lead time apparently posts an even greater challenge.)
- How can a company use information from web queries (similar to the browsing information used in Professor Provost’s research described above) to identify potential sales leads?
- When potential leads abound and resources available to respond to those leads are limited, can we develop a lead scoring system so that a company can properly filter out more important vs. less important leads?
- Different online marketing approaches use different types of content as input. For example, a company’s website and its social network presence most likely require different content. How can one measure the value of each content type to different segments and different industries?
- Demonstrate the ROI of social media efforts to help marketers argue the value of social media participation to upper-level managers.
In us academics’ constant quest for new knowledge, questions such as these are very useful in guiding our research effort toward being more relevant and applicable to practice. Here I send out a call to practitioners out there to supply us with more of these and to tell us the question marks in your head. Please feel free to leave your comment here. As the overarching goal for my blog, I would like to make Ping! an intersecting spot for practitioners and academic researchers.
This is going to be my last blog before Christmas. So here’s happy holidays to all my readers. Wish everyone a warm, safe and love-filled holiday!