For the longest time, I’ve wondered what brings the extraordinary success of some user-generated content. Consider, for example, the top ten most popular YouTube videos of all time. The #1 video on the list is a simple one-minute clip of a little baby biting his British English-accented brother’s finger. But it has received a whopping 155+ million views, while your average YouTube video probably doesn’t get much more than a dozen passerby’s attention. Why such a huge difference? I asked. When I spoke with my friend Michelle Rogerson, she expressed the same curiosity. So we decided to set out to answer our question.
To do this, we collected a random sample of slightly more than 100 videos from YouTube over the course of a week. These are all fresh new videos just uploaded onto YouTube, so that we can study their rise to popularity from scratch. We traced each video for a period of two months, recording the number of views and the average user ratings each day. We also collected a large number of characteristics for each video (see the figure below), including those related to the video content, to the video author, and to the network of users connected to the video author. We further recruited a group of individuals to rate each video on its production quality, educational value, and entertainment value, which are the three components of what we call “innate content quality”.
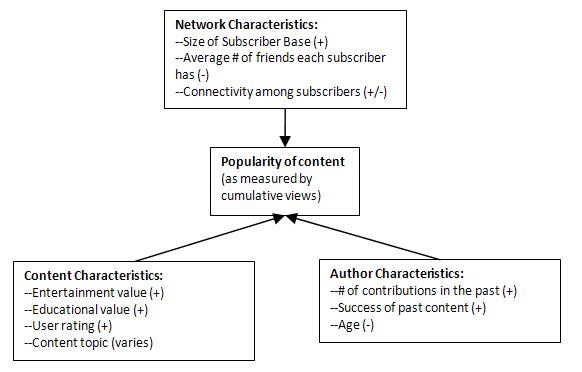
Equipped with all these data, we then used a technique called recurrent events analysis to see how these video characteristics affect the popularity of a video. Below are some of the main things we found:
- Authors with a large number of subscribers who each has only a handful of friends are in a better position than authors with a small number of subscribers who in turn may have a large number of friends.
- Once past an author’s direct network of subscribers, influence rather than simple reach becomes critical. We attribute this to the large amount of user-generated content (UGC) being passed around everyday and as a result our tendency to ignore most sharing unless they come from someone we are really close to or someone whose opinion we respect (opinion leadership is not dead!)
- What proportion of an author’s subscribers know each other also matters. A totally segregated set of subscribers does not help get the words out, but a group of subscribers where everyone knows everyone else is not optimal either. We found that the ideal connection ratio (termed connectivity or density in network analysis) was about 38%. Below this ratio, diffusion rate increases with connectivity up to the maximum, and then decreases after this threshold.
- Of the three innate quality components, entertainment value and educational value are equally important in determining a video’s popularity. Production quality, on the other hand, did not matter.
- But the biggest impact did not come from innate quality but from what we call “manifested quality”, which is quality information publicly available through other users’ ratings (i.e., the little stars underneath each YouTube video). Increasing the average rating by 1 star can lead to as much as 13.5% gain in diffusion rate.
- Age has a negative effect on diffusion rate, meaning that younger users’ contributions are more likely to be popular.
- An author’s past experience and success also carry over to the new content. More prolific authors and authors whose past contributions were more popular are more likely to see their new content popular.
Of course, with only one study, we are far from completely answering our initial question. But what we found here suggest that there are indeed systematic differences among videos and authors that can help predict the success of future content. Carrying this over to other types of user-generated content such as tweets and consumer blogs, these findings and findings from future studies should help companies pour through the overwhelming amount of user-generated content available online and selectively invest effort in the ones that are most likely to become popular.
What do you think? I’d love to hear your thoughts. Is there anything important that we are missing? If you are interested in more details about our study, you can download our working paper at http://www.yupingliu.com/files/papers/liu_rogerson_ugc_diffusion.pdf.