Since my recent series on building customer habits through customer relationship management, I have received a few inquiries about how we can figure out customers’ habit strength based on their transactional data. I will offer a brief explanation in this article. The approach I am discussing here assumes that you have individual customers’ purchase or product usage data available. If that’s not the case, you can refer to the last section for alternative measures of habit strength using customer surveys.
Two Key Components of Habit
To properly measure habit, we need to first understand what it is. In psychology and marketing, a habit is most commonly thought of as an action that is frequently repeated under the same situations. If we break this down, there are basically two ingredients to habit:
- Frequency: how frequently does someone take that action (e.g., watches TV, uses the mobile app, etc.)?
- Stability: when the person engages in the action, is it often under the same circumstances (e.g., on the way home, on Sunday morning, etc.)?
So to measure how strong a customer’s habit is, you need to find out about these two ingredients. The higher the frequency and the higher the stability, the stronger the habit is.
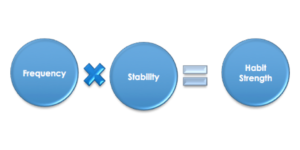
How to Measure Frequency
Measuring frequency is pretty straightforward. If you are dealing with purchase data, frequency means how many times on average someone buys your product in a given time window (e.g., per week, per month, per year, etc.). If you have usage data such as customer use of your mobile app, frequency means how many times someone uses the product in a given time length. Let’s say if someone buys your product 36 times a year, the average frequency per month would be 36/12 = 3 times.
While the concept is straightforward, you need to pay attention to what you choose as the time window for calculating your frequency measure. Let’s say your product is typically needed once a month or so, using a week as the time window would be too short to capture differences in the repeat patterns, because in most weeks people will not be buying anything. Instead, you’ll want to look at the monthly or quarterly level to have an idea of what the typical frequency is. On the other side of the coin, if your product is used very frequently, you don’t want your time window to be too wide either. For a product that is typically used daily, you don’t want your time window to be more than daily or weekly so that the frequency is not going to become too big of a number for the average customer.
How to Measure Stability
To measure how stable people’s behaviors are, you need to first decide what you consider as stable context in your setting. In an earlier post, I talked about the four typical types of contexts: time, location, social context, and preceding/subsequent event. In some published research, researchers have also considered purchase pattern factors such as how much the customer buys on promotion, buys low-margin products, and returns products purchased. You can also look into the content of what customers buy or use.
There are no hard and fast rules on what types of stability are the best ones to use. Here’re a couple of things that can help you pick the right ones:
- You can think about the typical behavior associated with your product and see what it is typically associated with. For example, if you run a coffee shop, time of the day is probably a big factor in your customers’ habit.
- You want to choose ones that differentiate your customers from each other. Say if you have a low-price strategy and attracts mostly customers who are promotion-minded, you may not want to use how much they buy on promotion as a stability cue, because it lacks the power to tell one customer apart from another.
- You may want to experiment with different stability dimensions and see which ones predict behavior the best using data analytics.
- Obviously your choice will be limited by the type of data you have. For example, if you do not capture time of use, you won’t be able to gauge stability on the time dimension.
Once you figure out the few stability elements you want to capture, the next step is to calculate the stability metric associated with each element. Let’s say you decided that time of day is an important stability component. That is, your strongly habitual customers would typically be using the product at the same time of the day (same in the sense of within the same person, not necessarily same across all your customers). Based on your business, you segment time of the day into five sections: early morning, mid-morning, lunch time, afternoon, evening, late night. For each customer, you’ll want to see what percentage of that customer’s purchases fall into each segment. Let’s say a customer’s purchase distribution is 90% early morning, 5% mid-morning, 3% lunch time, 2% afternoon, 0% for evening and late night. The time stability for this customer would be the highest of the percentages (in this case 90%). The rationale for this is fairly straightforward. If a customer tends not to purchase around the same time of day, you may see 20% of the customer’s purchases fall into each of the time segments. As a result, that customer’s stability would be 20% instead, much lower than the more habitual one with a 90% stability score.
This same calculation can be made with the other types of stability aspects such as location. Once you have a stability score for each stability dimension, you can create an overall stability score for each customer by averaging the customer’s stability scores across all dimensions. If a certain type of stability (e.g., time) is more important in your business than other stability dimensions (e.g., location), you may want to use a weighted average rather than a simple average.
Combining Frequency and Stability to Create a Habit Score
After you have figured out the frequency score and stability score for each customer, calculating habit is straightforward using the formula habit strength = frequency score x stability score. Since this is a very simple concept, I won’t dwell on this much. I do want to mention one technical detail. If your customers have wildly different frequencies (e.g., some with a frequency of 1 and some with a frequency of 200), in order for the frequency part not to over-dominate the habit metric, you’ll want to log-transform the frequency measure using transformed frequency score = log(frequency+1) (the plus 1 is to make sure those with zero frequency can still use the log-transformation). After this transformation, the 1 vs. 200 examples above will now be 0.69 vs. 5.3, a much more manageable range.
What If You Don’t Have Granulated Customer Data
As I mentioned at the beginning, the approach I described here is possible only if you have granulated customer-level behavioral data. If you don’t have that information, it is still possible to gauge your customers’ habit strength. But you’ll have to rely on good ol’ self-reported measures using customer surveys. The downside of this approach is that because habit is supposed to follow an automatic process, people may not be fully aware that they have a strong habit and therefore may not rate themselves very high on these measures even if they are strongly habitual. In my experience of using different habit metrics, these self-reported measures tend to correlate with the behavior-based measures at a moderate level (correlations in the 0.3~0.4 range).
Below are two sources where you’ll find self-reported habit measures:
- “Habits in Everyday Life: Thought, Emotion, and Action” by Wood, Quinn and Kashy, published in 2002 in Journal of Personality and Social Psychology, Vol. 83, No. 6, p.1281-1297. (Habit measure details are on p.1285)
- “Reflections on Past Behavior: A Self-Report Index of Habit Strength” by Verplanken and Orbell, published in 2003 in Journal of Applied Social Psychology, Vol. 33, No. 6, p.1313-1330.
I hope this article helps you solve the technical challenge of identifying the habitual individuals among your customers. It’s a bit more technical than my typical writing. So if you need further help after reading the article, please feel free to reach out to me. Thank you for reading!